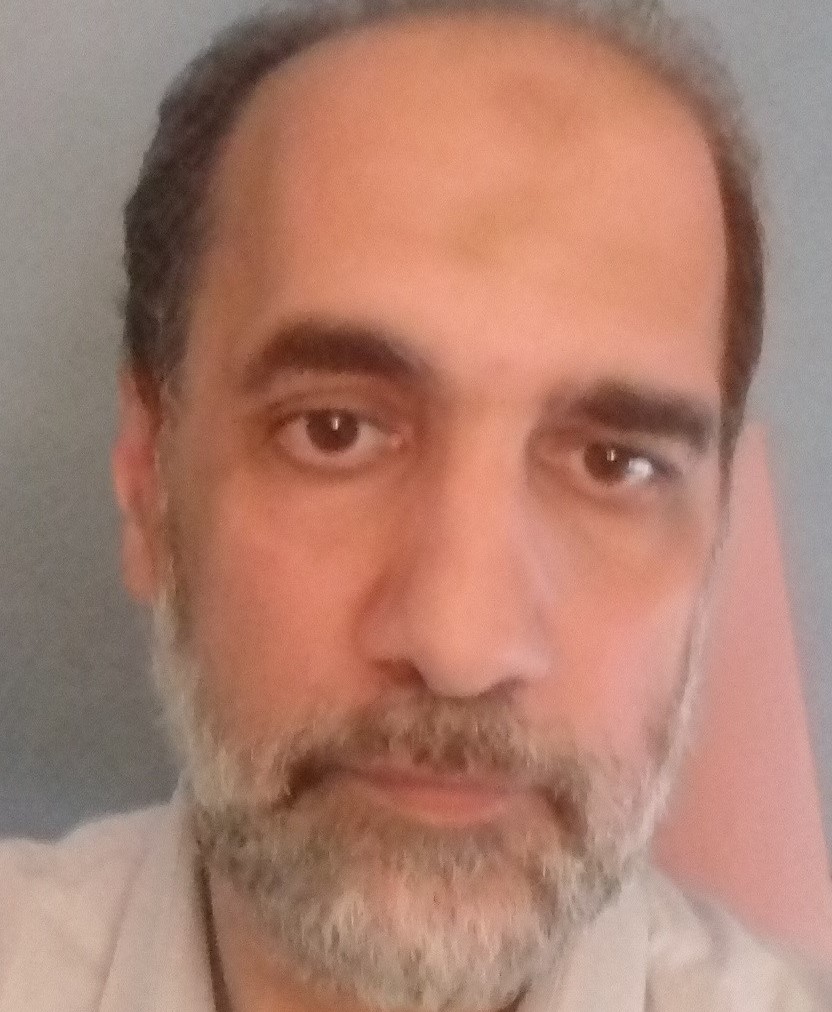
PRISME / University of Orléans, INSA CVL - FR
He was born in 1967. He received the State Engineering Degree from the École Polytechnique, Palaiseau, France, in 1990, the State Engineering Degree from the École Nationale Supérieure des Télécommunications (ENST), Paris, France, in 1992, the M.Sc. degree from Paris XI University, Orsay, France, in 1992, and the Ph.D. degree in the field of signal processing and communications from ENST, in 1995. From 1995 to 1998, he was a Research Staff with the Electrical Engineering Department, The University of Melbourne, where he worked on several research project related to “Blind System Identification for Wireless Communications”, “Blind Source Separation,” and “Array Processing for Communications.” From 1998 to 2012, he has been an Assistant Professor, then an Associate Professor with the Signal and Image Processing Department, Télécom ParisTech. In September 2012, he joined the University of Orléans, France (PRISME Laboratory), as a Full Professor. His research interests include signal processing for communications, adaptive filtering and tracking, array processing, and statistical performance analysis. He is the author of about 500 scientific publications, including book chapters, international journal and conference papers, and patents. Dr. Abed-Meraim is currently a member of the IEEE SAM-TC and a Senior Area Editor of the IEEE Transactions on Signal Processing.
Tensor methods: Concepts, Algorithms & Applications
The era of “Big Data”, which deals with massive datasets, has brought new analysis techniques for discovering new valuable information hidden in the data. Among these techniques is multilinear low-rank approximation (LRA) of matrices and tensors, which has recently attracted a lot of attention from engineers and researchers in the signal processing and machine learning communities. A tensor is a multidimensional array and provides a natural representation of high-dimensional data. Low-rank approximation of tensors (t-LRA) can be considered as a multiway extension of LRA of matrices (which are two-way) to higher dimensions. Generally, t-LRA is referred to as tensor decomposition which allows factorizing a tensor into a sequence of basic components. As a result, t-LRA provides a useful tool for dealing with several large-scale multidimensional problems in modern data analysis which would be, otherwise, intractable by classical methods.
This lecture is a brief review of different tensor concepts and different tensor decomposition algorithms with illustrative application examples in biomedical signal processing. It is addressed to a wide audience with general background in signal processing.