I got my Master Degree in Biomedical Engineering at Politecnico di Milano in 2018. I’m currently a PhD candidate in Bioengineering at Politecnico di Milano, under the supervision of Prof. Francesco Migliavacca, and I will defend my thesis in early 2023. The topic of my research is the development of computational models of the treatments for stroke, from high-fidelity finite-elements models, to reduced order models. Part of my research was carried out within the EU Horizon2020 project INSIST (IN Silico trials for treatment of acute Ischemic STroke). In July 2022 I received the Young Researcher Prize at the European Solid Mechanics Conference in Galway (Ireland) with a work on the investigation of the impact of thrombus composition on the outcome of thrombectomy procedures.
Dimensionality reduction and kernel optimization for the prediction of thrombectomy outcomes
Endovascular thrombectomy (EVT) is the main treatment for acute ischemic stroke, aiming at removing the thrombus from a cerebral artery with a stent-retriever. The treatment must be performed in the first few hours from symptoms onset, allowing a short time window for pre-operative planning. Computational simulations of EVT can predict the treatment outcome but require a long computational time. In this work, a classification model is proposed, trained on high-fidelity EVT simulations, for providing fast estimates on the success of the procedure. The training dataset is composed of 94 finite-elements patient-specific EVT simulations, containing cases with successful (thrombus removed) and unsuccessful (thrombus not removed) outcomes, which are highly unbalanced: 81 successful against 13 unsuccessful EVTs. The proposed strategy is made of two steps. First, a dimensionality reduction approach is used to parametrize the vascular anatomies with few dimensions. Then, given as inputs the anatomy description and the thrombus properties for each virtual patient, a kernel function is trained, based on a restricted semidefinite positive optimization, with which clusters are created according to the EVT success. With the obtained tool, once data describing a new patient is available, it is processed through the trained kernel so that the results may approach either the successful or the unsuccessful cluster, suggesting a higher or lower probability of success of a possible EVT intervention.
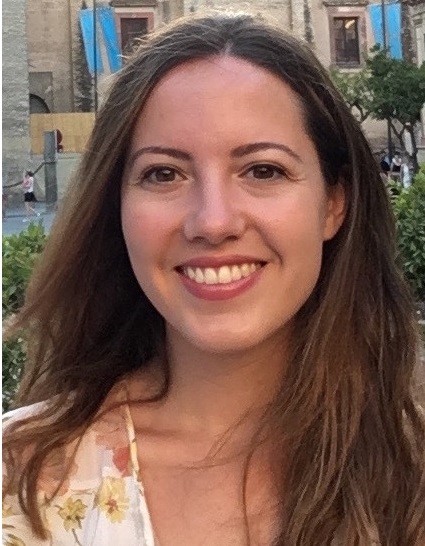
Politecnico di Milano
Piazza Leonardo da Vinci 32
Milano - IT
Email: sara.bridio@polimi.it
Tel.: +390223993399