Prof. Wen Chen
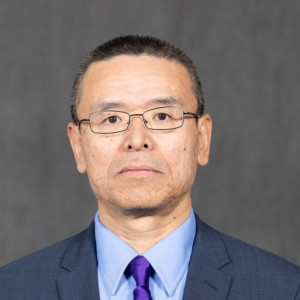
From
In residence at
PRISME / INSA Centre Val de Loire, University of Orléans - FR
Host scientist
Prof. Driss Boutat
BIOGRAPHY
Dr. Wen Chen received his PhD degree from Simon Fraser University, Burnaby, British Columbia, Canada, in 2004 and pursued his postdoctoral research at University of Louisiana at Lafayette from 2005 to 2007. He became a control systems engineer in 2008 and joined Wayne State University in 2009 as an assistant professor. Currently, he is a full professor with tenure. His research interests have been in the area of Control systems and Fault diagnosis, including control, estimation, and diagnosis of Lithium-ion batteries, etc. Dr. Chen has performed various funded projects supported by the Department of Energy, National Science Foundation, Michigan state government, and industrial companies, etc. He has published over 130 articles in prestigious journals and refereed conferences. He won Teaching Awards in Division of Engineering Technology for many years (2011-2021), and a Teaching Award of College of Engineering in 2013. Currently, he is the Chair of Faculty Assembly Executive Committee of College of Engineering at Wayne State University (2024-2026).
PROJECT
Fault Diagnosis of Networked Battery Systems for Industrial Risk Control and Management
The goal of the research in this project is to investigate and advance a new mechanism that could contribute to industrial risk control and management of networked battery systems using a new fault diagnosis technology towards practical applications. New approaches to diagnosing faults and/or failures of networked battery systems will be developed for networked battery systems with string terminal measurements. The following three aims will be performed. Aim 1: Develop a switching scheme of cell-balancing circuits to meet the needs of observability for fault diagnosis without affecting the capability of cell balancing in real time. Aim 2: To investigate and further advance a new observer-based fault-diagnostic scheme for Lithium-ion batteries, a fractional-order battery model will be constructed. Aim 3: A new fractional-order learning observer will be advanced for fault diagnostic purposes.